Solve Systems of Nonlinear Equations in MATLAB with scipy.optimize.root()
Part 9 of the Python Is The Ultimate MATLAB Toolbox series.
Introduction
Systems of nonlinear equations appear in many diciplines of
science, engineering, and economics1.
Examples include
mechanical linkages,
power flow,
heat transfer,
and
Calvo pricing.
MATLAB’s Optimization Toolbox
has functions that can solve such equations.
If you don’t have access to this toolbox and you’re willing to
include Python in your MATLAB workflow,
you can use SciPy’s
scipy.optimize.root()
to find solutions
to systems of nonlinear equations in MATLAB.
- Example: The GPS Equations
- Defining the function to solve
- GPS Equations Solved in Python
- GPS Equations Solved in MATLAB
Example: The GPS Equations
Satellites in the global positioning system (GPS) constellation continuously transmit a navigation message which includes information about their orbital positions and the precise time. A GPS receiver that can get signals from at least four GPS satellites can compute its location by solving the nonlinear algebraic equations defining the intersection of four spheres, where the spheres' radii are determined by how long it took the messages to reach the receiver while traveling at the speed of light. Technically, only three spheres are needed to resolve two points in space, one of which can typically be rejected as a candidate based on its distance from the earth’s surface. However, since GPS receivers' clocks are inaccurate compared to the atomic clocks on the satellites, using a fourth satellite provides an extra equation that lets us additionally solve for the receiver’s clock error.
The four spheres problem can actually be simplified to a system of linear equations which is much easier to solve. For the sake of this post, however, the nonlinear equations are solved directly to demonstrate how such problems are set up in Python and MATLAB.
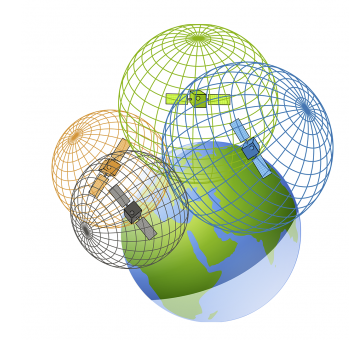
The equations for the four spheres are
where numeric subscripts denote the satellite; $A$, $B$, and $C$ are earth-centered coordinates (such as ECI or ECEF) of each satellite; $t$ is the time when the satellite sent its navigation message; $T_R$ is the receiver’s time; $e$ is the error in the receiver’s clock; and $c$ is the speed of light, 299,792.458 km/s. These values come from the GMU article:
satellite | $A_i$ [km] | $B_i$ [km] | $C_i$ [km] | $\Delta_i = t_i - T_R$ [seconds] |
---|---|---|---|---|
1 | 15600 | 7540 | 20140 | 0.07074 |
2 | 18760 | 2750 | 18610 | 0.07220 |
3 | 17610 | 14630 | 13480 | 0.07690 |
4 | 19170 | 610 | 18390 | 0.07242 |
We’re solving for the receiver’s position, ($x$, $y$, $z$), and the error in its clock, $e$. Our location ($x$, $y$, $z$) will be in the same coordinate system, ECEF or ECI, used to define ($A$, $B$, $C$) and $e$ will be in seconds.
Defining the function to solve
scipy.optimize.root()
’s primary arguments are the function to solve
and a starting guess. In this context, “solve” means to find values
for the function’s inputs so that the function returns zeros for
all outputs, in other words, to find the roots of the function.
To this end we’ll rewrite our equations so they equal zeros
then write a Python function that returns the four computed values
of the left hand sides:
The input variables $(x, y, z, e)$
need to be stored in a list or NumPy array.
We’ll use the Python variable x
as a four item array so that
$(x, y, z, e)$ are stored in x[0]
through x[3]
.
The function also needs each satellite’s position, $(A_i, B_i, C_i)$,
and the time difference between the satellite’s clock and the
receiver’s clock, $t_i - T_R$ which we’ll store as Dt
:
Python
# file: gps_location.py
def F(x, A, B, C, Dt):
c_light = 299792.458 # km/s
return (
(x[0]-A[0])**2 + (x[1]-B[0])**2 + (x[2]-C[0])**2 - (c_light*(Dt[0] + x[3]))**2,
(x[0]-A[1])**2 + (x[1]-B[1])**2 + (x[2]-C[1])**2 - (c_light*(Dt[1] + x[3]))**2,
(x[0]-A[2])**2 + (x[1]-B[2])**2 + (x[2]-C[2])**2 - (c_light*(Dt[2] + x[3]))**2,
(x[0]-A[3])**2 + (x[1]-B[3])**2 + (x[2]-C[3])**2 - (c_light*(Dt[3] + x[3]))**2,
)
We’ll also call this function from MATLAB so it needs to be
in a file we can import.
The examples below, both Python and MATLAB, will use F()
from the file
gps_location.py
.
GPS Equations Solved in Python
We can solve for our position and clock error by populating arrays with satellite data then calling SciPy’s multivariate root finder with a starting guess. The earth’s radius is about 6378 km so a reasonable guess would be a random point near the earth’s surface, for example (6000, 0, 0). The clock error should be much less than a second so we’ll use 0 as a guess for that term.
The Python function we’re finding roots of, F()
, takes four
additional arguments, namely the three arrays of satellite position, and
time deltas, in addition to the input vector x
.
These additional arguments can be passed through to F()
by giving
them to scipy.optimize.root()
via the args
keyword option:
Python
#!/usr/bin/env python
import numpy as np
import scipy.optimize
from gps_function import F
A = np.array([15600, 18760, 17610, 19170.0]) # km/s
B = np.array([ 7540, 2750, 14630, 610.0]) # km/s
C = np.array([20140, 18610, 13480, 18390.0]) # km/s
Dt = np.array([0.07074, 0.07220, 0.07690, 0.07242]) # s
guess = [6000, 0, 0, 0]
location = scipy.optimize.root(F, guess, args=(A, B, C, Dt))
print(location)
The output includes a text string indicating whether or not
the solution converged (.message
), the number of times the function
was evaluated (.nfev
), the $4 \times 4$ Jacobian matrix (.fjac
)
and the 4 terms of function at the computed solution (.fun
).
The solution itself is in the return variable’s .x
attribute:
fjac: array([[-0.42964552, -0.5286645 , -0.49262911, -0.54151189],
[ 0.20220688, -0.32707893, 0.75636015, -0.52919852],
[ 0.8684367 , -0.05711611, -0.42770859, -0.24417374],
[-0.14260014, 0.78119842, -0.0479713 , -0.60588199]])
fun: array([-1.19209290e-07, -5.96046448e-08, -1.19209290e-07, -5.96046448e-08])
message: 'The solution converged.'
nfev: 14
qtf: array([ 3.35605879e-05, -1.62470220e-06, -2.25947450e-06, 2.73577863e-07])
r: array([ 6.38261526e+04, 2.49011922e+04, 3.81328894e+04, 2.78876134e+10,
-2.27583853e+04, 4.74674593e+03, -1.98003469e+09, -1.01001959e+04,
-1.33002639e+09, 2.38095380e+08])
status: 1
success: True
x: array([-4.17727096e+01, -1.67891941e+01, 6.37005956e+03, 3.20156583e-03])
(-1.1920928955078125e-07, -5.960464477539063e-08, -1.1920928955078125e-07, -5.960464477539063e-08)
From location.x
we see that our GPS receiver is near the North Pole
at (-41.772, -16.789, 6370.060) km
and its clock is off by 3.20156583e-03 seconds.
location.fun
has the function’s value at the solution. Since all values
should be zero, this array represents the solution’s error.
GPS Equations Solved in MATLAB
MATLAB can call scipy.optimize.root()
as easily as Python can,
provided that the function we’re finding the roots of is
implemented in Python.
(This is the same restriction we encountered minimizing a function
in MATLAB
with scipy.optimize.differential_evolution()
.)
In our case we’ll reuse the function F()
from gps_function.py
:
MATLAB 2020b+
% file: solve_gps.m
so = py.importlib.import_module('scipy.optimize');
gps = py.importlib.import_module('gps_function');
A = [15600, 18760, 17610, 19170.0]; % km/s
B = [ 7540, 2750, 14630, 610.0]; % km/s
C = [20140, 18610, 13480, 18390.0]; % km/s
Dt = [0.07074, 0.07220, 0.07690, 0.07242]; % s
guess = [6000, 0, 0, 0];
ABCDt = py.tuple({A, B, C, Dt});
location = so.root(gps.F, guess, pyargs('args',ABCDt))
The location
found in MATLAB is the same as the one found
in Python:
>> solve_gps
location =
Python OptimizeResult with no properties.
fjac: array([[-0.42964552, -0.5286645 , -0.49262911, -0.54151189],
[ 0.20220688, -0.32707893, 0.75636015, -0.52919852],
[ 0.8684367 , -0.05711611, -0.42770859, -0.24417374],
[-0.14260014, 0.78119842, -0.0479713 , -0.60588199]])
fun: array([-1.19209290e-07, -5.96046448e-08, -1.19209290e-07, -5.96046448e-08])
message: 'The solution converged.'
nfev: 14
qtf: array([ 3.35605879e-05, -1.62470220e-06, -2.25947450e-06, 2.73577863e-07])
r: array([ 6.38261526e+04, 2.49011922e+04, 3.81328894e+04, 2.78876134e+10,
-2.27583853e+04, 4.74674593e+03, -1.98003469e+09, -1.01001959e+04,
-1.33002639e+09, 2.38095380e+08])
status: 1
success: True
x: array([-4.17727096e+01, -1.67891941e+01, 6.37005956e+03, 3.20156583e-03])
Note the object’s class: Python OptimizeResult
. Extracting
values from such an object is possible but clumsy as the return values are also
Python objects:
>> format long e
>> location.get('x')
ans =
Python ndarray:
-4.177270957085472e+01 -1.678919410652270e+01 6.370059559223343e+03 3.201565829594195e-03
Use details function to view the properties of the Python object.
Use double function to convert to a MATLAB array.
We’d much prefer a native MATLAB struct. That’s easily done
with
py2mat.m
2:
>> loc = py2mat(location)
struct with fields:
x: [-4.177270957085472e+01 -1.678919410652270e+01 6.370059559223343e+03 3.201565829594195e-03]
success: 1
status: 1
nfev: 14
fjac: [4×4 double]
r: [6.382615261849605e+04 2.490119219503466e+04 3.813288936837602e+04 2.788761343528719e+10 … ]
qtf: [3.356058790625163e-05 -1.624702195475986e-06 -2.259474496258976e-06 2.735778627939572e-07]
fun: [-1.192092895507812e-07 -5.960464477539062e-08 -1.192092895507812e-07 -5.960464477539062e-08]
message: "The solution converged."
Table of Contents | Previous: Solve global optimization problems | Next : Symbolic math
-
Wolfgang Eichhorn & Winfried Gleißner, 2016. “Nonlinear Functions of Interest to Economics. Systems of Nonlinear Equations,” Springer Texts in Business and Economics, in: Mathematics and Methodology for Economics, edition 1, chapter 7, pages 301-371, Springer. ↩︎
-
I added support for
Python OptimizeResult
objects inpy2mat.m
on 2022-09-16. Earlier versions will fail to convert such objects. ↩︎